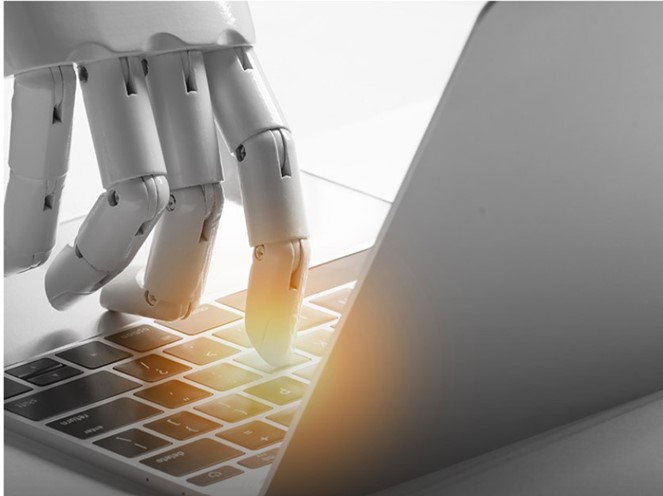
Will AI Learn to Become a Better Entrepreneur than You?
Contemporary businesses use artificial intelligence (AI) tools to assist with operations and compete in the marketplace. AI enables firms and entrepreneurs to make data-driven decisions and to quicken the data-gathering process. When creating strategy, buying, selling, and increasing marketplace discovery, firms need to ask: What is better, artificial or human intelligence?
A recent article from the Harvard Business Review, “Can AI Help You Sell?,” stated, “Better algorithms lead to better service and greater success.” The attributes of the successful entrepreneur, such as calculated risk taking, dealing with uncertainty, keen sense for market signals, and adjusting to marketplace changes might be a thing of the past. Can AI take the place of the human entrepreneur? Would sophisticated artificial intelligence be able to spot market prices better, adjust to expectations better, and steer production toward the needs of consumers better than a human?
In one of my classes this semester, students and I discussed the role of AI, deep machine learning, and natural language processing (NLP) in driving many of the decisions and operations a human would otherwise provide within the firm. Of course, half of the class felt that the integration of some level of AI into many firms’ operations and resource management is beneficial in creating a competitive advantage.
However, the other half felt using AI will inevitably disable humans’ function in the market economy, resulting in less and less individualism. In other words, the firm will be overrun by AI. We can see that even younger college students are on the fence about whether AI will eliminate humans’ function in the market economy. We concluded as a class that AI and machine learning have their promises and shortcomings.
After class, I started thinking about the digital world of entrepreneurship. E-commerce demands the use of AI to reach customers, sell goods, produce goods, and host exchange—in conjunction with a human entrepreneur, of course.
However, AI—machine learning or deep machine learning—could also be tasked with creating a business-based model, examining the data on customers’ needs, designing a web page, and creating ads. Could AI adjust to market action and react to market uncertainty like a human? The answer may be a resounding yes! So, could AI eliminate the human entrepreneur?
Algorithm-XLab explains deep machine learning as something that “allows computers to solve complex problems. These systems can even handle diverse masses of unstructured data set.” Algorithm-XLab compared deep learning with human learning favorably, stating, “While a human can easily lose concentration, and possibly make a mistake, a robot won’t.”
This statement by Algorithm-XLab challenges the idea that trial and error leads to greater market knowledge and better enables entrepreneurs to provide consumers with what they are willing to buy. The statement also portrays the marketplace as a process where people have perfect knowledge and an equilibrium point, and it implies that humans do not have specialized knowledge of time and place.
The use of AI and its tools of deep learning and language processing do have their benefits from a technical standpoint. AI can determine how to produce hula hoops better, but can it determine whether to produce them or devote energy elsewhere? If entrepreneurs discover market opportunities, they must weigh the advantages and disadvantages of their potential actions. Will AI have the same entrepreneurial foresight?
The acquisition of market knowledge can take humans years to acquire; AI is much faster at it than humans would be. For example, the Allen Institute for AI is “working on systems that can take science tests, which require a knowledge of unstated facts and common sense that humans develop over the course of their lives.” The ability to process unstated, scattered facts is precisely the kind of characteristic we attribute to entrepreneurs. Processes, changes, and choices characterize the operation of the market, and the entrepreneur is at the center of this market function.
There is no doubt that contemporary firms use deep learning for strategy, operations, logistics, sales, and record keeping for human resources (HR) decision-making, according to a Bain & Company article titled “HR’s New Digital Mandate.” While focused on HR, the digital mandate does lend itself to questioning the use of entrepreneurial thinking and strategy conducted within a firm. After AI has learned how to operate a firm using robotic process automation and NLP capacities to their maximum, might it outstrip the human natural entrepreneurial abilities?
AI is used in everyday life, such as self-checkout at the grocery store, online shopping, social media interaction, dating apps, and virtual doctor appointments. Product delivery, financing, and development services increasingly involve an AI-as-a-service component. AI as a service minimizes the costs of gathering and processing customer insights, something usually associated with a team of human minds projecting key performance indicators aligned with an organizational strategy.
The human entrepreneur has a competitive advantage insofar as handling ambiguous customer feedback and in effect creating an entrepreneurial response and delivering satisfaction. We seek to determine whether AI has replaced human energy in some areas of life. Can AI understand human uneasiness or dissatisfaction, or the subjectivity of value felt by the consumer? AI can produce hula hoops, but can it articulate plans and gather the resources needed to produce them in the first place?.
In what, if any, entrepreneurial functions can AI outperform the human entrepreneur? The human entrepreneur is willing to take risks, adjust to the needs of consumers, pick up price signals, and understand customer choices. Could the human entrepreneur soon become an extinct class? If so, would machine learning and natural processing AI understand the differences between free and highly regulated markets? If so, which would it prefer, or which would it create?