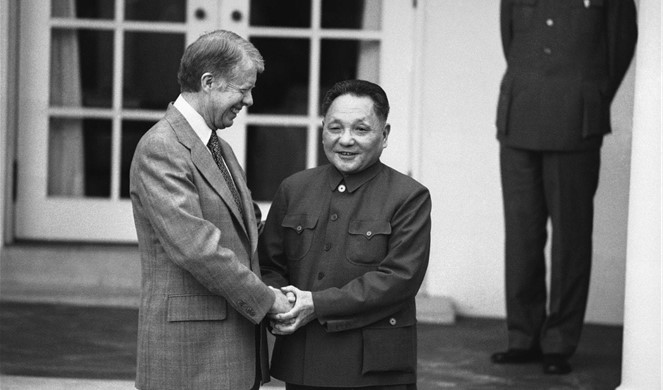
The US and China May Be Ending an Agreement on Science and Technology Cooperation − A Policy Expert Explains What This Means for Research
A decades-old science and technology cooperative agreement between the United States and China expires this week. On the surface, an expiring diplomatic agreement may not seem significant. But unless it’s renewed, the quiet end to a cooperative era may have consequences for scientific research and technological innovation.
The possible lapse comes after U.S. Rep. Mike Gallagher, R-Wis., led a congressional group warning the U.S. State Department in July 2023 to beware of cooperation with China. This group recommended to let the agreement expire without renewal, claiming China has gained a military advantage through its scientific and technological ties with the U.S.
The State Department has dragged its feet on renewing the agreement, only requesting an extension at the last moment to “amend and strengthen” the agreement.
The U.S. is an active international research collaborator, and since 2011 China has been its top scientific partner, displacing the United Kingdom, which had been the U.S.‘s most frequent collaborator for decades. China’s domestic research and development spending is closing in on parity with that of the United States. Its scholastic output is growing in both number and quality. According to recent studies, China’s science is becoming increasingly creative, breaking new ground.
This article was republished with permission from The Conversation, a news site dedicated to sharing ideas from academic experts. It represents the research-based findings and thoughts of, Caroline Wagner, Professor of Public Affairs, The Ohio State University.
As a policy analyst and public affairs professor, I research international collaboration in science and technology and its implications for public policy. Relations between countries are often enhanced by negotiating and signing agreements, and this agreement is no different. The U.S.’s science and technology agreement with China successfully built joint research projects and shared research centers between the two nations.
U.S. scientists can typically work with foreign counterparts without a political agreement. Most aren’t even aware of diplomatic agreements, which are signed long after researchers have worked together. But this is not the case with China, where the 1979 agreement became a prerequisite for and the initiator of cooperation.
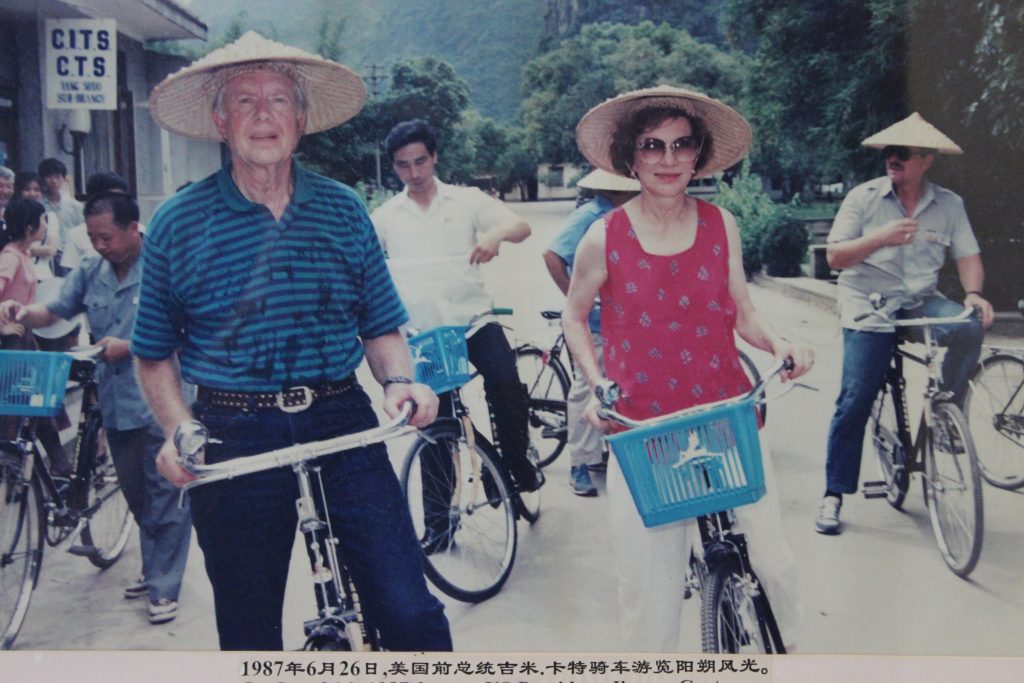
A 40-Year Diplomatic Investment
The U.S.-China science and technology agreement was part of a historic opening of relations between the two countries, following decades of antagonism and estrangement. U.S. President Richard Nixon set in motion the process of normalizing relations with China in the early 1970s. President Jimmy Carter continued to seek an improved relationship with China.
China had announced reforms, modernizations and a global opening after an intense period of isolation from the time of the Cultural Revolution from the late 1950s until the early 1970s. Among its “four modernizations” was science and technology, in addition to agriculture, defense and industry.
While China is historically known for inventing gunpowder, paper and the compass, China was not a scientific power in the 1970s. American and Chinese diplomats viewed science as a low-conflict activity, comparable to cultural exchange. They figured starting with a nonthreatening scientific agreement could pave the way for later discussions on more politically sensitive issues.
On July 28, 1979, Carter and Chinese Premier Deng Xiaoping signed an “umbrella agreement” that contained a general statement of intent to cooperate in science and technology, with specifics to be worked out later.
In the years that followed, China’s economy flourished, as did its scientific output. As China’s economy expanded, so did its investment in domestic research and development. This all boosted China’s ability to collaborate in science – aiding their own economy.
Early collaboration under the 1979 umbrella agreement was mostly symbolic and based upon information exchange, but substantive collaborations grew over time.
A major early achievement came when the two countries published research showing mothers could ingest folic acid to prevent birth defects like spina bifida in developing embryos. Other successful partnerships developed renewable energy, rapid diagnostic tests for the SARS virus and a solar-driven method for producing hydrogen fuel.
Joint projects then began to emerge independent of government agreements or aid. Researchers linked up around common interests – this is how nation-to-nation scientific collaboration thrives.
Many of these projects were initiated by Chinese Americans or Chinese nationals working in the United States who cooperated with researchers back home. In the earliest days of the COVID-19 pandemic, these strong ties led to rapid, increased Chinese-U.S. cooperation in response to the crisis.

Time of Conflict
Throughout the 2000s and 2010s, scientific collaboration between the two countries increased dramatically – joint research projects expanded, visiting students in science and engineering skyrocketed in number and collaborative publications received more recognition.
As China’s economy and technological success grew, however, U.S. government agencies and Congress began to scrutinize the agreement and its output. Chinese know-how began to build military strength and, with China’s military and political influence growing, they worried about intellectual property theft, trade secret violations and national security vulnerabilities coming from connections with the U.S.
Recent U.S. legislation, such as the CHIPS and Science Act, is a direct response to China’s stunning expansion. Through the CHIPS and Science Act, the U.S. will boost its semiconductor industry, seen as the platform for building future industries, while seeking to limit China’s access to advances in AI and electronics.
A Victim of Success?
Some politicians believe this bilateral science and technology agreement, negotiated in the 1970s as the least contentious form of cooperation – and one renewed many times – may now threaten the United States’ dominance in science and technology. As political and military tensions grow, both countries are wary of renewal of the agreement, even as China has signed similar agreements with over 100 nations.
The United States is stuck in a world that no longer exists – one where it dominates science and technology. China now leads the world in research publications recognized as high quality work, and it produces many more engineers than the U.S. By all measures, China’s research spending is soaring.
Even if the recent extension results in a renegotiated agreement, the U.S. has signaled to China a reluctance to cooperate. Since 2018, joint publications have dropped in number. Chinese researchers are less willing to come to the U.S. Meanwhile, Chinese researchers who are in the U.S. are increasingly likely to return home taking valuable knowledge with them.
The U.S. risks being cut off from top know-how as China forges ahead. Perhaps looking at science as a globally shared resource could help both parties craft a truly “win-win” agreement.